The role of machine learning in automating financial operations
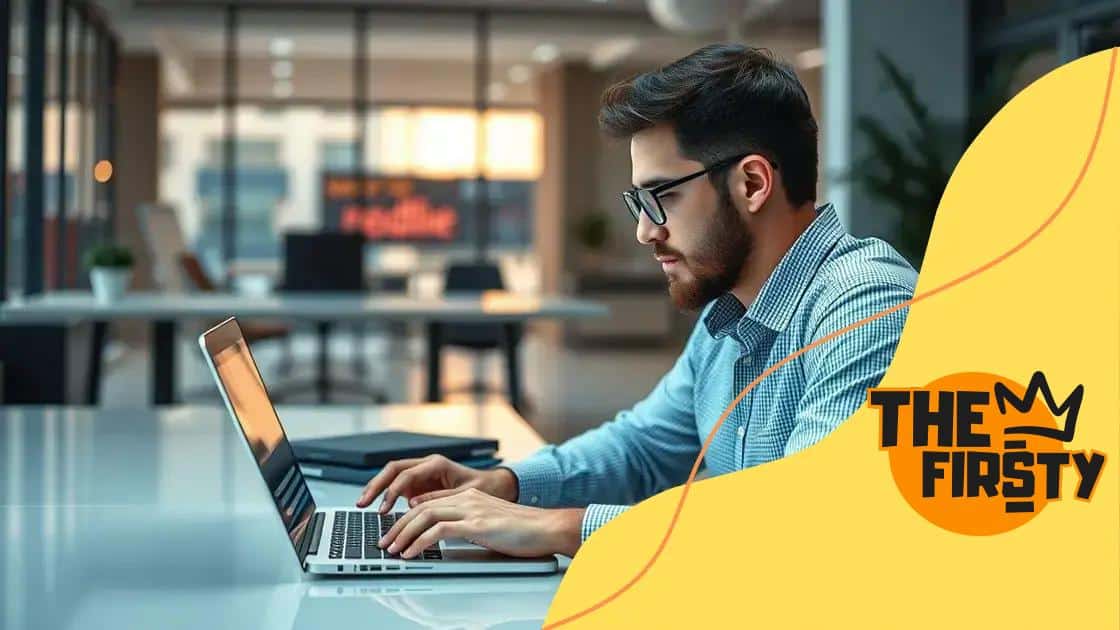
The role of machine learning in automating financial operations enhances efficiency, reduces costs, and improves risk management through advanced data analysis and decision-making capabilities.
The role of machine learning in automating financial operations is becoming increasingly vital. By simplifying complex tasks, businesses can improve accuracy and save time. How does this technology change the financial landscape?
Understanding machine learning in finance
Understanding machine learning in finance is essential for grasping how technology is changing the industry. This innovative approach helps analyze vast data, making predictions and decisions faster than traditional methods. As such, businesses gain a competitive edge.
What is Machine Learning?
Machine learning refers to the ability of computers to learn from data without being explicitly programmed. It uses algorithms to identify patterns and make decisions based on input data.
Applications in Finance
In finance, machine learning transforms how data is analyzed. It can enhance risk assessment, for instance. Here are some key applications:
- Fraud detection: Machine learning algorithms analyze transaction patterns to identify unusual activities.
- Credit scoring: They evaluate credit risk more accurately by examining various data points.
- Algorithmic trading: Traders use machine learning to predict stock price movements and optimize trading strategies.
- Customer insights: Financial institutions can personalize services by understanding customer behavior through data analysis.
These applications show how finance can benefit significantly from machine learning. Automating and improving these processes leads to better decision-making.
Furthermore, the adoption of machine learning encourages financial entities to adapt to market changes promptly. For example, models can adjust to new trends, greatly enhancing the relevant insight.
Challenges and Considerations
Though the potential of machine learning in finance is remarkable, challenges remain. Data quality is crucial; poor data can lead to inaccurate predictions. Additionally, institutions need to address regulatory compliance when implementing machine learning solutions.
In conclusion, understanding machine learning in finance is vital for leveraging its advantages. As financial operations increasingly rely on technology, embracing this shift will be crucial for success in the future. Financial professionals must keep pace with these changes to enhance their strategies and services.
Key benefits of automation
Automation in finance offers numerous advantages that can greatly transform the industry. By leveraging technology, companies can streamline operations and enhance efficiency across various processes.
Increased Efficiency
One of the main benefits of automation is improved efficiency. Automated systems can handle repetitive tasks quickly and accurately, allowing employees to focus on more complex and valuable activities.
Cost Savings
Another significant advantage is cost savings. By reducing manual labor and minimizing errors, organizations can lower their operational costs. Here are some detailed benefits:
- Labor costs: Automation reduces the need for extensive human resources in certain tasks.
- Fewer errors: Machines perform calculations with precision, leading to fewer costly mistakes.
- Faster processes: Speeding up operations translates to increased productivity and profitability.
- Resource optimization: Companies can allocate their resources more effectively, directing attention to growth activities.
Through this strategic use of resources, companies can see a noticeable boost in profit margins. Automation also helps enhance accuracy in financial reporting and data analysis.
Improved Compliance
In the highly regulated finance sector, automation aids in ensuring compliance with regulations. Automated systems can monitor transactions in real time, flagging any discrepancies that require attention. This reduces the risk of non-compliance and associated penalties.
Moreover, reliable automation provides a clear audit trail for all financial activities. This makes it easier for organizations to adhere to regulations and facilitates smoother audits when necessary.
Enhanced Customer Experience
Improving customer experience is another critical benefit of automation. With faster processing times, clients receive timely responses to their inquiries. Automation can also personalize services, offering tailored suggestions to meet individual customer needs.
As businesses implement automated solutions, they can enhance service quality and establish stronger relationships with clients.
Use cases of machine learning in finance
Machine learning has numerous use cases in finance that showcase its transformative power. By analyzing data patterns, financial institutions can significantly improve their services and operations.
Fraud Detection
One of the most critical applications of machine learning is in fraud detection. Financial institutions utilize algorithms to monitor transaction patterns and identify unusual activities that may indicate fraud. These systems learn from historical data, continually improving their accuracy in recognizing potential threats.
Risk Assessment
Risk assessment is another vital area where machine learning proves beneficial. By analyzing customer data, organizations can evaluate credit risk more accurately. Machine learning models can consider various factors beyond traditional credit scores, leading to better lending decisions. Key features include:
- Real-time analysis: Instant access to data allows for swift decision-making.
- Enhanced predictive capabilities: Models can evolve with changing data trends.
- More informed decisions: Accurate risk profiles help mitigate potential losses.
This sophisticated analysis ensures financial entities can minimize risks while maximizing profits.
Algorithmic Trading
Machine learning also plays a significant role in algorithmic trading. By processing large datasets, it helps traders identify patterns and trends that inform their strategies. Traders can execute orders at optimal times and improve their overall performance. Here’s how:
- Data analysis: ML systems analyze stock movements and market conditions.
- Speed: Real-time data processing enables quick market reactions.
- Reduced emotional bias: Automated systems focus solely on data, avoiding human error.
The result is more efficient and profitable trading strategies.
Customer Service
Another important use case is enhancing customer service. With chatbots powered by machine learning, financial institutions can provide real-time assistance to customers. These chatbots can handle inquiries, recommend products, and improve overall client satisfaction. The benefits are notable:
- 24/7 availability: Clients can receive support at any time.
- Personalization: Services can be tailored based on customer preferences.
- Efficiency: Reduces wait times for assistance.
This leads to happier clients and more engaged users.
Challenges in implementing machine learning
Implementing machine learning in finance comes with its set of challenges. While the benefits are significant, businesses must navigate obstacles to achieve successful integration.
Data Quality and Availability
One major challenge is ensuring data quality and availability. Machine learning models require large amounts of accurate data to function effectively. If the data is incomplete, outdated, or inaccurate, the models may produce unreliable results. Many companies struggle with:
- Data silos: Different departments may hold valuable data, but it often remains isolated and underutilized.
- Lack of standardization: Inconsistent data formats can complicate the merging and analysis of information.
- Data privacy concerns: Navigating regulations can limit access to sensitive information.
Improving data management practices is essential for overcoming these hurdles and enhancing model performance.
Technical Expertise
Another challenge is the requirement for technical expertise. Machine learning projects demand skilled professionals who can develop, implement, and maintain these systems. Finding qualified personnel can be difficult, especially in a competitive job market. Companies often face:
- Limited talent pool: The need for specialized skills can create hiring challenges.
- Training and development: Existing staff may require upskilling to meet new demands.
- Retention of expertise: High turnover can result in lost institutional knowledge.
Organizations must invest in training programs while also fostering a workplace that attracts and retains talent.
Integration with Legacy Systems
Integrating machine learning with existing legacy systems poses another significant challenge. Many financial institutions rely on older technologies that may not seamlessly connect with newer solutions. This can create:
- Compatibility issues: Older systems may have difficulty processing modern data formats.
- Increased costs: Upgrading infrastructure can require substantial financial investment.
- Disruption of services: Transitioning to new systems without proper planning can lead to outages.
Effectively managing these changes is crucial for a smooth transition.
Change Management
Lastly, managing change within the organization can prove challenging. Employees may fear job displacement due to automation. Ensuring proper communication and training can help alleviate these concerns. It’s important to:
- Engage employees: Involve staff in the transition to emphasize their value.
- Provide support: Offer resources to help employees adapt to new technologies.
- Foster a culture of innovation: Encourage experimentation and learning with new tools.
Addressing these issues proactively paves the way for a smoother implementation process.
Future trends in financial operations
The future of financial operations is evolving rapidly, driven by technological advancements and changing market dynamics. Key trends are reshaping how businesses operate, making them more efficient and customer-focused.
Increased Automation
One of the most significant trends is the increase in automation across financial processes. Companies are leveraging automation to streamline operations, reduce costs, and improve accuracy. As tasks like data entry and transaction processing become automated, employees can focus on higher-value work such as strategy and analysis.
AI and Machine Learning Integration
The integration of artificial intelligence (AI) and machine learning continues to grow in the finance sector. These technologies can analyze vast amounts of data to uncover insights and make informed decisions. Some of the specific benefits include:
- Predictive analytics: Financial institutions can forecast market trends based on historical data.
- Risk management: ML models help assess risks more accurately, enabling better decision-making.
- Customer personalization: AI-driven recommendations provide tailored financial solutions.
This shift not only boosts efficiency but also enhances customer experience through tailored services.
Blockchain Technology
Blockchain is another trend gaining traction in financial operations. Its ability to enable secure, transparent transactions is revolutionizing how financial data is managed. Benefits of blockchain implementation include:
- Improved security: The decentralized nature makes it harder for malicious attacks to occur.
- Faster transactions: Blockchain can reduce processing times from days to minutes.
- Cost reductions: Lower transaction fees result from removing intermediaries.
As these technologies evolve, they are expected to create new opportunities for growth.
Cloud Computing Adoption
Cloud computing is transforming the way financial institutions manage data and deliver services. The shift to cloud-based systems allows for greater flexibility and scalability. Key advantages of cloud adoption include:
- Accessibility: Employees can access information from anywhere, promoting remote work.
- Cost efficiency: Reduced need for physical infrastructure and maintenance costs.
- Enhanced collaboration: Teams can work together more effectively with real-time data access.
This trend is particularly important as businesses aim to adapt to a more digital landscape.
Regulatory Technology (RegTech)
As regulations become more complex, the demand for regulatory technology, or RegTech, is on the rise. This involves using technology to help companies comply with regulations efficiently. Benefits include:
- Automation of compliance tasks: Streamlining the process of meeting regulatory requirements.
- Real-time monitoring: Continuous oversight to quickly address compliance issues.
- Data analytics for compliance: Analyzing large datasets to ensure adherence to laws and regulations.
By embracing RegTech, organizations can navigate the regulatory landscape with ease.
FAQ – Frequently Asked Questions about Machine Learning in Finance
What are the main benefits of automation in finance?
Automation in finance improves efficiency, reduces costs, and enhances accuracy by streamlining repetitive tasks.
How does machine learning help in fraud detection?
Machine learning analyzes transaction patterns to identify anomalies, thereby preventing fraudulent activities in real-time.
What role does blockchain play in financial operations?
Blockchain enhances security and transparency in transactions, reducing the chances of fraud and increasing trust among parties.
Why is data quality important for machine learning models?
High-quality data is essential as it ensures accurate predictions and reliable outcomes from machine learning models, impacting decision-making.